Flume calibration on irrigated systems by Video Image Processing and Bayesian Inference
DOI:
https://doi.org/10.31285/AGRO.27.1182Keywords:
flume calibration, RIveR, BaRatinAgeAbstract
Rice is a crop that requires a large amount of water throughout its production cycle to ensure a good yield, resulting in higher water consumption compared to other crops. In Uruguay, about 160,000 ha/year are planted, requiring about 1,760 hm3/year of water, with a very high international average productivity of 9,000 kg/ha. Irrigation is generally carried out by surface/flooding, with water passing through dug channels where gates are used to regulate the flow, and in some cases measurement devices are installed. Increasing pressure on water resources makes it necessary to increase knowledge of water use at the farm level. Flumes are an opportunity in this sense; however, they require calibration and adjustment through gauging, which are generally omitted due to their high cost and complexity. In this work, an economic method for the calibration of flumes through image video processing is proposed. The method uses the RIveR software (https://riverdischarge.blogspot.com/) for the video image processing, and the BaRatinAGE software to establish the stage-discharge relationship through Bayesian inference. A Surface Velocity Radar and an Acoustic Doppler Velocity Meter are used as reference sensors. The methodology is tested on a cutthroat flume. The experiment was conducted at a rice farm in northern Uruguay. The results indicate that flumes can be easily calibrated by video image processing and uncertainty can be quantified through Bayesian inference. An advantage of the proposed method is that it uses free software that can be easily applied in small farms.
Downloads
References
Adeogun A, Mohammed A. Review of methods of measuring streamflow using hydraulic structures. En: Ahsan A, editor. Hydraulic structures: theory and applications [Internet]. London: IntechOpen; 2019 [citado 2023 Aug 21]. 12p. Disponible en: https://cdn.intechopen.com/pdfs/64627.pdf
Atkins MD. Velocity field measurement using Particle Image Velocimetry (PIV). En: Kim T, Lu TJ, Song SJ, editores. Application of thermo-fluidic measurement techniques: an introduction. Amsterdam: Elsevier; 2016. pp. 125¬66. Doi: 10.1016/B978-0-12-809731-1.00005-8.
Benacchio V, Piégay H, Buffin-Bélanger T, Vaudor L. A new methodology for monitoring wood fluxes in rivers using a ground camera: potential and limits. Geomorphology. 2017;279:44-58.
Berger JO, Pericchi LR. Training samples in objective Bayesian model selection. Ann Stat. 2004;32(3):841-69.
Betancur L, Ruiz A, Castaño V, Duran P. Medición de agua y transmisión de datos en sistemas de riego por gravedad. En: Arroz: resultados experimentales 2015-2016. Montevideo: INIA; 2016. p. 23-5.
Bi S, Bai Y, Zhou X. Bayesian updating: reducing epistemic uncertainty in hysteretic degradation behavior of steel tubular structures. ASCE ASME J Risk Uncertain Eng. 2022;8(3):04022039. Doi: 10.1061/AJRUA6.000125.
Carracelas G, Hornbuckle J, Rosas J, Roel A. Irrigation management strategies to increase water productivity in Oryza sativa (rice) in Uruguay. Agric Water Manag. 2019;222:161-72.
Catsamas S, Shi B, Deletic B, Wang M, McCarthy DT. A low-cost, low-power water velocity sensor utilizing acoustic doppler measurement. Sensors (Basel). 2022;22(19):7451. Doi: 10.3390/s22197451.
Cawley KM, Harrison N, Nickerson ZL. NEON user guide to stage‐discharge rating curves (NEON.DP4.00133) [Internet]. [lugar desconocido]: NEON; 2022 [citado 2023 Aug 21]. 14p. Disponible en: https://data.neonscience.org/api/v0/documents/NEON_ratingCurve_userGuide_vC?inline=true
Dobriyal P, Badola R, Tuboi C, Hussain SA. A review of methods for monitoring streamflow for sustainable water resource management. Appl Water Sci. 2017;7(6):2617-28.
Ferdous R, Khan F, Sadiq R, Amyotte P, Veitch B. Handling and updating uncertain information in bow-tie analysis. J Loss Prev Process Ind. 2012;25(1):8-19.
Fujita I, Muste M, Kruger A. Large-scale particle image velocimetry for flow analysis in hydraulic engineering applications. J Hydraul Res. 1998;36(3):397-414.
Garcia R, Costa V, Silva F. Bayesian rating curve modeling: alternative error model to improve low-flow uncertainty estimation. J Hydrol Eng. 2020;25(5):04020012. Doi: 10.1061/(ASCE)HE.1943-5584.0001903.
Green PJ, Łatuszyński K, Pereyra M, Robert CP. Bayesian computation: a summary of the current state, and samples backwards and forwards. Stat Comput. 2015;25(4):835-62.
Guía de buenas prácticas en el cultivo de arroz en Uruguay [Internet]. Montevideo: ACA; 2009 [citado 2023 Aug 21]. 32p. Disponible en: http://www.ainfo.inia.uy/digital/bitstream/item/4927/1/Guia-de-Buenas-Practicas-Arroz-Uruguay-ACA.pdf
Hauet A. Estimation de débit et mesure de vitesse en rivière par Large-scale particle image velocimetry. Houille Blanche. 2009;(1):80-5. Doi: 10.1051/lhb:2009009.
Hauet A, Morlot T, Daubagnan L. Velocity profile and depth-averaged to surface velocity in natural streams: a review over alarge sample of rivers. E3S Web Conf. 2018;40:06015. Doi: 10.1051/e3sconf/20184006015.
Heiner B. Parshall flume staff gauge location and entrance wingwall discharge calibration corrections [tesis de maestría]. Logan (US): Utah State University, Civil and Environmental Engineering; 2009. 46p. Doi: 10.26076/eae6-ebf8.
Heyrani M, Mohammadian A, Nistor I, Dursun OF. Application of numerical and experimental modeling to improve the efficiency of parshall flumes: a review of the state-of-the-art. Hydrology. 2022;9(2):26. Doi: 10.3390/hydrology9020026.
Horner I, Renard B, Le Coz J, Branger F, McMillan HK, Pierrefeu G. Impact of stage measurement errors on streamflow uncertainty. Water Resour Res. 2018;54(3):1952-76.
Jolley MJ, Russell AJ, Quinn PF, Perks MT. Considerations when applying large-scale piv and ptv for determining river flow velocity. Front Water. 2021;3:709269. Doi: 10.3389/frwa.2021.709269.
Kastali A, Zeroual A, Remaoun M, Serrano-Notivoli R, Moramarco T. Design flood and flood-prone areas under rating curve uncertainty: area of Vieux-Ténès, Algeria. J Hydrol Eng. 2021;26(3):05020054. Doi: 10.1061/(ASCE)HE.1943-5584.0002049.
Kazimierski LD, García PE, Ortiz N, Morales M. Aforos de ríos y arroyos en la Cuenca Matanza-Riachuelo: Informe 05. Elaboración de relaciones altura – caudal (curvas HQ) [Internet]. Ezeiza: Acumar; 2021 [citado 2023 Aug 21]. 163p. Disponible en: https://repositorio.ina.gob.ar/items/fc1504ca-a46c-4c6a-99cd-68184626bcd4
Khosronejad A, Herb W, Sotiropoulos F, Kang S, Yang X. Assessment of Parshall flumes for discharge measurement of open-channel flows: a comparative numerical and field case study. Measurement. 2021;167:108292. Doi: 10.1016/j.measurement.2020.108292.
Kraatz DB, Mahajan IK, editores. Small hydraulic structures [Internet]. Vol. 2. Rome: FAO; 1975 [citado 2023 Aug 21]. 292p. Disponible en: https://www.fao.org/3/bl046e/bl046e.pdf
Le Coz J. A literature review of methods for estimating the uncertainty associated with stage-discharge relations [Internet]. Geneva: WMO; 2012 [citado 2023 Aug 21]. 21p. Report No.: PO6a:21. Disponible en: https://citeseerx.ist.psu.edu/document?repid=rep1&type=pdf&doi=b685243d91acd17a64c3e31ecff08ea39d5b279d
Le Coz J, Jodeau M, Hauet A, Marchand B, Le Boursicaud R. Image-based velocity and discharge measurements in field and laboratory river engineering studies using the free FUDAA-LSPIV software. En: River Flow 2014 [Internet]. Lausanne: [editor desconocido]; 2014 [citado 2023 Aug 21]. pp. 7. Disponible en: https://hal.inrae.fr/hal-02600735
Lüthi B, Philippe T, Peña-Haro S. Mobile device app for small open-channel flow measurement. En: Ames DP, Quinn NWT, Rizzoli AE, editores. Proceedings of the 7th International Congress on Environmental Modelling and Software, June 15-19, San Diego, California, USA [Internet]. [lugar desconocido]: IEMSS; 2014 [citado 2023 Aug 21]. pp. 2837. Available from: https://scholarsarchive.byu.edu/cgi/viewcontent.cgi?article=1035&context=iemssconference
Mansanarez V, Le Coz J, Renard B, Lang M, Pierrefeu G, Vauchel P. Bayesian analysis of stage-fall-discharge rating curves and their uncertainties. Water Resour Res. 2016;52(9):7424-43.
Martin J, Elster C. Aleatoric uncertainty for errors-in-variables models in deep regression. Neural Process Lett. 2022;55:4799-818. Doi: 10.1007/s11063-022-11066-3.
Muste M, Xiong Z, Schöne J, Li Z. Validation and extension of image velocimetry capabilities for flow diagnostics in hydraulic modeling. J Hydraul Eng. 2004;130(3):175-85.
Navas R, Vervoort W, Gamazo P. Bayesian inference of synthetic daily rating curves by coupling Chebyshev polynomials and the GR4J model. En: IAHS Scientific Assembly 2022. [lugar desconocido]: IAHS; 2022. Doi: 10.5194/iahs2022-2.
Patalano A, García CM, Rodríguez A. Rectification of Image Velocity Results (RIVeR): a simple and user-friendly toolbox for large scale water surface Particle Image Velocimetry (PIV) and Particle Tracking Velocimetry (PTV). Comput Geosci. 2017;109:323-30.
Porter NW, Mousseau VA. Understanding aleatory and epistemic parameter uncertainty in statistical models. En: Best Estimate Plus Uncertainty International Conference [Internet]. 2020 [citado 2023 Aug 21]. 10p. Disponible en: https://www.osti.gov/servlets/purl/1811616
Ran D, Wang W, Hu X. Three-dimensional numerical simulation of flow in trapezoidal cutthroat flumes based on FLOW-3D. Front Agr Sci Eng. 2018;5(2):168-76. Doi: 10.15302/J-FASE-2018217.
Rozos E, Mazi K, Lykoudis S. On the accuracy of particle image velocimetry with citizen videos: five typical case studies. Hydrology. 2022;9(5):72. Doi: 10.3390/hydrology9050072.
Rubio PB, Chamoin L, Louf F. Real-time Bayesian data assimilation with data selection, correction of model bias, and on-the-fly uncertainty propagation. Comptes Rendus Mécanique. 2019;347(11):762-79.
Sanjou M, Kato K, Aizawa W, Okamoto T. Development of drone-type float for surface-velocity measurement in rivers. Environ Fluid Mech. 2022;22(4):955-69.
Skogerboe GV, Bennett RS, Walker WR. Generalized discharge relations for cutthroat flumes. J Irrig Drain Div. 1972;98(4):569-83.
Temeepattanapongsa S, Merkley GP, Barfuss SL, Smith BL. Generic free-flow rating for cutthroat flumes. J Hydraul Eng. 2013;139(7):727-35.
Tomas G, Bleninger T, Rennie C, Guarneri H. Advanced 3D mapping of hydrodynamic parameters for the analysis of complex flow motions in a submerged bedrock canyon of the Tocantins River, Brazil. Water. 2018;10(4):367. Doi: 10.3390/w10040367.
Wasserman L. All of statistics: a concise course in statistical inference. Pittsburgh: Springer; 2004. 442p.
World Meteorological Organization. Manual on stream gauging. Geneva: WMO; 2010. 2p.
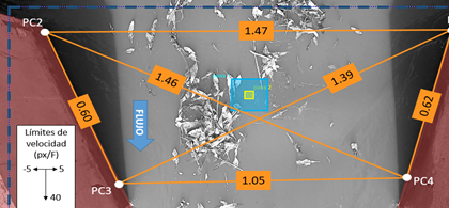
Downloads
Published
How to Cite
Issue
Section
License
Copyright (c) 2023 Agrociencia Uruguay

This work is licensed under a Creative Commons Attribution 4.0 International License.
Article metrics | |
---|---|
Abstract views | |
Galley vies | |
PDF Views | |
HTML views | |
Other views |